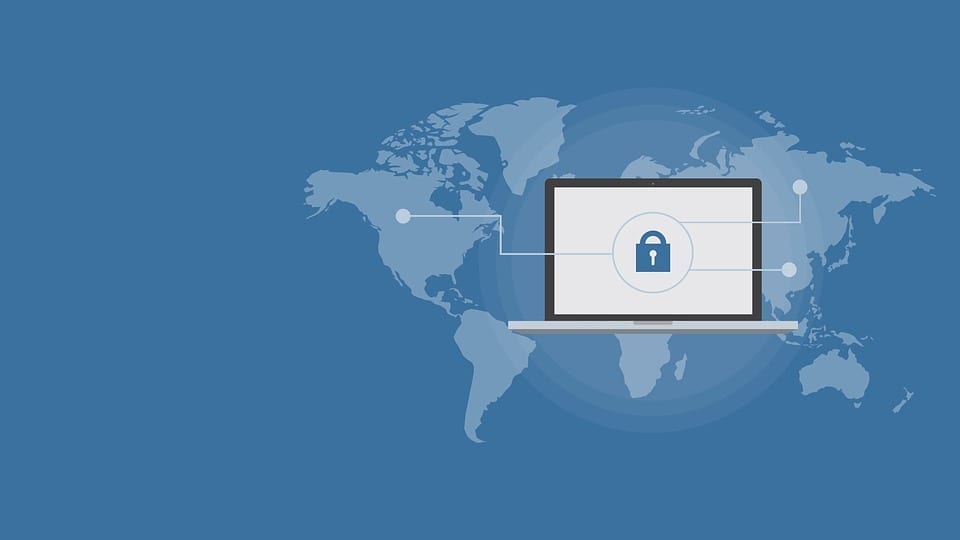
Big data is the base for the next revolution in the field of Information Technology. Companies today irrespective of their size are making huge investments in the field of big data analytics. This traction comes because of the obvious competitive advantages that data provides in today’s market landscape. Consider this, for a typical Fortune 1000 company, a 10% increase in available data can translate to more than $65 million increase in the net income. Unfortunately, companies have largely been unsuccessful in making sense out of data. According to a NewVantage Partners survey, only 37.1% of the companies surveyed believe that they were successful in their big data endeavor. Hence, it is important to understand the prominent big data challenges and the steps you should take to overcome them.
Interested in Big Data Analytics? Checkout our eBook on latest innovations, use cases and developments:
Top 5 Big Data Challenges and their Viable Solutions:
1. Handling Voluminous Data:
The rate at which big data is generated is outpacing the development of computing and storage systems. According to IDC report by 2020, the amount of data will be sufficient to fill a stack of tablets equivalent to 6.6 times the distance between the earth and the moon. Unsurprisingly managing unstructured data is becoming more and more challenging - 31%in 2015 to 45% in 2016 - as reported by analysts.
Adding to this is the rise of complex data formats like audio, video, documents, social media, and the new smart devices. As per the IDC report, online business transactions will reach up to 450 billion per day. According to a research by Cisco, the number of connected devices will reach 50 billion in just 5 years, all of these will generate a massive amount of data.
We have now resorted to supplementing RDBMS (Relational databases) with more dynamic NoSQL Databases like MongoDB and DynamoDB. Obviously storing data is not the end goal. We also need to analyze it and generate insights. To handle the computation and analysis many distributed computing systems like Hadoop, Hive, Pig etc. are being used by organizations.
Read More: 3 Reasons: Why Most Big Data Projects Fail?
2. Shortage of Data Scientists:
The thinking of data scientists and business leaders is hardly ever on the same page. Entry-level analysts usually deviate from the genuine business value of data and end up with insights that don’t really solve the problem in question. On top of that is the limited number of data sciences available in the market who can deliver value.
2017 Robert Half Technology Salary Guide revealed that all professionals in the field of big data were paid exceptionally well. Still, organizations face difficulties in retaining top talent and training entry-level technicians from scratch is expensive.
Many organizations, as a result, are developing self-service analysis solutions that use automation, machine learning, and artificial intelligence to make sense out of data with minimal manual coding. For the time being, we can see a general upward trend in recruitment and training budget till the problem is solved.
Read More: Why Choose Python for a Big Data Project?
3. Getting Real-time Insights:
A great deal of complexity is added when we move from analyzing stagnant data to handling real-time inputs. This requires a new range of data analysis tools that can handle data of high veracity and velocity. These tools include ETL engines, visualization, computation, frameworks, and libraries.
Business often loses on important relevant information because of failure to keep pace with the rapidly originating data. This doesn’t come as a surprise that the PwC's Global Data and Analytics Survey 2016 found, "Everyone wants decision-making to be faster.” This is especially true for banking, insurance, and healthcare.
Recent innovations like AWS Glue - a fully automated Extract, Transform and Load (ETL) engine have eased the job of data scientists but there is still a long way to go. There also some other tools in this space like Xplenty, StarfishETL etc. each of these has distinct offerings but overall aim to ease ETL, integrations and database management. In future, we will see more sophisticated tools to make things easier.
Read More: The 7 Step Big Data Strategy
4. Data Governance and Security:
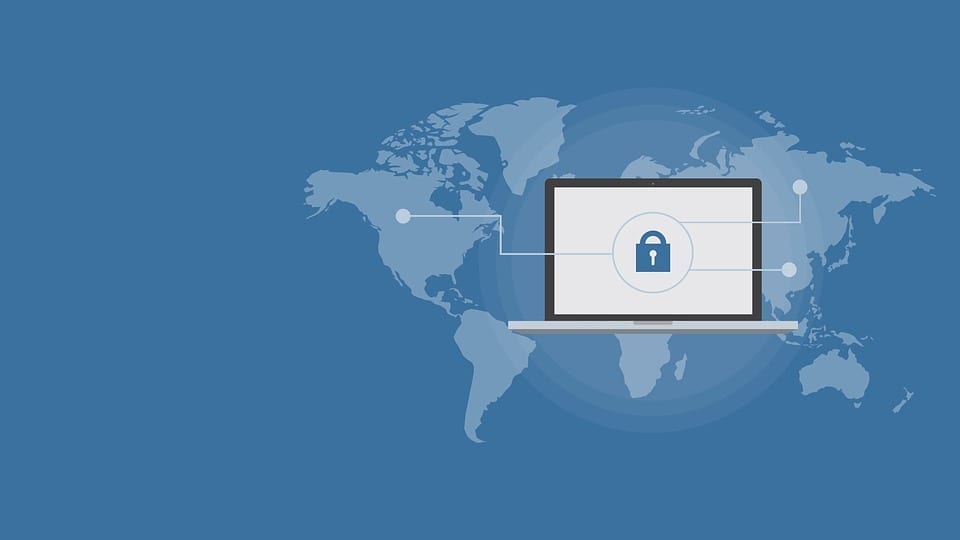
Big data involves dealing with data from multiple sources and most of these sources use a distinct format and collection method for their data. Hence it is common to find inconsistencies in data for the value of the same variable and adjusting for them is very challenging. For instance, in a retail business, the value of annual turnover can be different according to the local POC, online sales tracker, enterprise resource planning (ERP) and company accounts. In such a scenario, the difference needs to be adjusted to find the appropriate answer. This process is called data governance.
Another problem associated with data is ensuring its security and integrity. Since the number of channels and interconnecting nodes is high the chances of hackers exploiting a vulnerability in the system increase multifold. Due to the criticality of the data, a minor accident can result in huge losses hence companies are bound to introduce the best security practices in their systems.
Companies are actively using advanced solutions that leverage machine learning to fight cybercrimes. One of the popular example here the Amazon Macie - a cloud-based service that intelligently discovers, classifies and protects critical data in the AWS architecture. With the increase in demand for such solutions, we will see many innovations in this space.
Read More: 6 Hacks to Ensure Data Security
5. Organizational Resistance:
Building a database architecture in the company is not just the matter of hiring some data scientists. In fact, that is the easiest part as you can simply outsource the analysis. The most prominent challenge is pivoting the company’s architecture, structure and culture to implement data-based decision making.
Among the top challenges faced by leaders today are insufficient organizational alignment, lack of adoption and understanding of the middle management and business resistance. Making these changes is very challenging for large enterprises who have built and scaled their operations on the traditional mechanisms.
According to PWC experts, companies need to hire strong leaders who not just understand data but also take the initiative to challenge the status quo of the current practices and suggest relevant changes. According to a survey by NewVantage Partners, 55.9 % of Fortune 1000 companies have hired Chief Data Officers (CDOs) to meet this requirement.
If you are looking for people who can leverage big data amidst all these challenges then you’re at the right place. With a skilled team of data scientists and passion for new age technologies, we are ready to handle any level of complex problems persistent in this field. Contact us today for a POC or consultation.